Table Of Content
In other words, when the error term is inflated, the percentage of variability explained by the statistical model diminishes. Therefore, the model becomes a less accurate representation of reality. So if you don’t block, you will reduce the statistical power of the study. So, the block to block variability is then absorbed in the variance estimator of the residual. Hence, the power gain of the RCB is a trade-off between the variability that can be explained by the block effect and the loss in DF.
4 Experimental Design III: Randomized Complete Block Designs and Pseudo-replication
A non-blocked way to run this experiment would be to run each of the twelve experimental wafers, in random order, one per furnace run. That would increase the experimental error of each resistivity measurement by the run-to-run furnace variability and make it more difficult to study the effects of the different dosages. The blocked way to run this experiment, assuming you can convince manufacturing to let you put four experimental wafers in a furnace run, would be to put four wafers with different dosages in each of three furnace runs. The only randomization would be choosing which of the three wafers with dosage 1 would go into furnace run 1, and similarly for the wafers with dosages 2, 3 and 4. Implementing blocking in experimental design involves a series of steps to effectively control for extraneous variables and enhance the precision of treatment effect estimates. So what types of variables might you need to balance across your treatment groups?
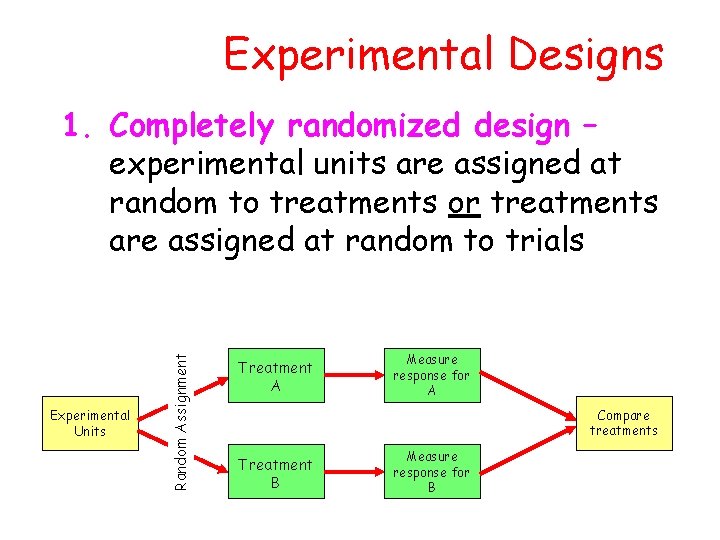
Random Allocation
We could select the first three columns - let's see if this will work. Click the animation below to see whether using the first three columns would give us combinations of treatments where treatment pairs are not repeated. However, this method of constructing a BIBD using all possible combinations, does not always work as we now demonstrate. If the number of combinations is too large then you need to find a subset - - not always easy to do. However, sometimes you can use Latin Squares to construct a BIBD. As an example, let's take any 3 columns from a 4 × 4 Latin Square design.
When should you use blocking?
Blocking can also be understood as replicating an experimenton multiple sets, e.g., different locations, of homogeneous experimental units,e.g., plots of land at an individual location. The experimental units shouldbe as similar as possible within the same block, but can be very differentbetween different blocks. This design allows us to fully remove thebetween-block variability, e.g., variability between different locations, fromthe response because it can be explained by the block factor. In that sense, blocking is a so-calledvariance reduction technique. The use of blocking in experimental design has an evolving history that spans multiple disciplines. The foundational concepts of blocking date back to the early 20th century with statisticians like Ronald A. Fisher.
Randomized Complete Block Design Analysis Model
A Latin Square design blocks on both rows and columnssimultaneously. If we ignore the columns of a Latin Square designs, the rows form anRCBD; if we ignore the rows, the columns form an RCBD. Here we have two pairs occurring together 2 times and the other four pairs occurring together 0 times. Therefore, this is not a balanced incomplete block design (BIBD). Randomized Complete Block Design (RCBD) is arguably the most common design of experiments in many disciplines, including agriculture, engineering, medical, etc. In addition to the experimental error reducing ability, the design widens the generalization of the study findings.
A condensate-hardening drug blocks RSV replication in vivo - Nature.com
A condensate-hardening drug blocks RSV replication in vivo.
Posted: Wed, 07 Jul 2021 07:00:00 GMT [source]
This kind of design is used to minimize the effects of systematic error. If the experimenter focuses exclusively on the differences between treatments, the effects due to variations between the different blocks should be eliminated. This website is using a security service to protect itself from online attacks. There are several actions that could trigger this block including submitting a certain word or phrase, a SQL command or malformed data. The next thing you need to do after you determine your blocking factors is allocate your observations into blocks. To simplify things, we will assume that you have one main blocking factor that you want to balance over.
We cannot fit a more complex model, includinginteraction effects, here because we do not have the corresponding replicates. Hence, a block is given by a locationand an experimental unit by a plot of land. In the introductory example, a blockwas given by an individual subject. Why is it important to make sure that the number of soccer players running on turf fields and grass fields is similar across different treatment groups? In this article we tell you everything you need to know about blocking in experimental design. First we discuss what blocking is and what its main benefits are.
To assess the effectiveness of two different ways of teaching reading, a group of 5-year-olds was recruited from a primary school. Their level of reading ability was assessed, and then they were taught using scheme one for 20 weeks. To assess the difference in reading comprehension between 7 and 9-year-olds, a researcher recruited each group from a local primary school.
Lesson 4: Blocking
We consider an example which is adapted from Venables and Ripley (2002), the original source isYates (1935) (we will see the full data set in Section 7.3). Atsix different locations (factor block), three plots of land were available.Three varieties of oat (factor variety with levels Golden.rain, Marvellousand Victory) were randomized to them, individually per location. Typical block factors are location (see example above), day (if an experiment isrun on multiple days), machine operator (if different operators are needed forthe experiment), subjects, etc. Often, the researcher is not interested in the block effect per se, but he only wants to account for the variability in response between blocks. Of note, the block effect is typically considered as a random effect. Finally, if you expect the 'treatment effect' to differ from block to block, then interactions should be considered.
More specifically, blocking is used when you have one or more key variables that you need to ensure are similarly distributed within your different treatment groups. A matched pairs design is an experimental design where pairs of participants are matched in terms of key variables, such as age or socioeconomic status. One member of each pair is then placed into the experimental group and the other member into the control group. Independent measures design, also known as between-groups, is an experimental design where different participants are used in each condition of the independent variable. This means that each condition of the experiment includes a different group of participants.
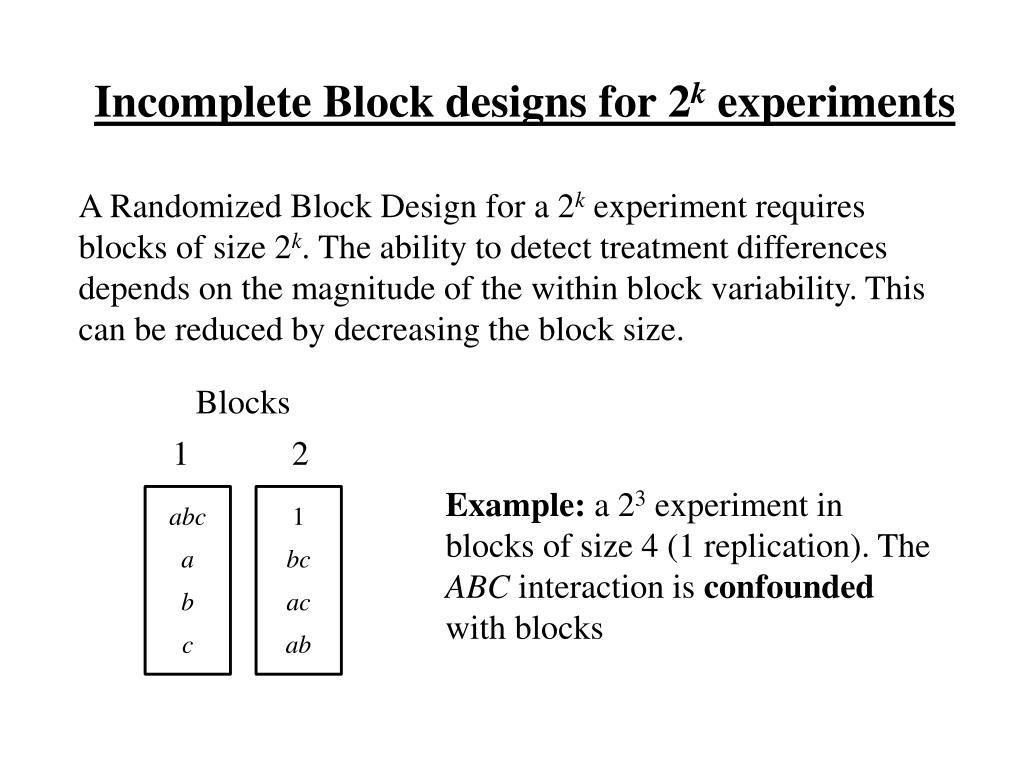
The Design Structure has one factor (oven run, Run), and the Treatment Structure two factors (Recipe and Temperature). Because every run has to be a single (nominal) temperature, Temperature and Run must occur at the same level of the experimental design. So far we have discussed experimental designs with fixed factors, that is, the levels of the factors are fixed and constrained to some specific values. In some cases, the levels of the factors are selected at random from a larger population. In this case, the inference made on the significance of the factor can be extended to the whole population but the factor effects are treated as contributions to variance. In general, we are faced with a situation where the number of treatments is specified, and the block size, or number of experimental units per block (k) is given.
They were given the same passage of text to read and then asked a series of questions to assess their understanding. To compare the effectiveness of two different types of therapy for depression, depressed patients were assigned to receive either cognitive therapy or behavior therapy for a 12-week period. When in doubt, decide on the number of blocks based on previous literature.
No comments:
Post a Comment